Data quality and quantity: open contracting needs both to work well. So what are we going to do about it?
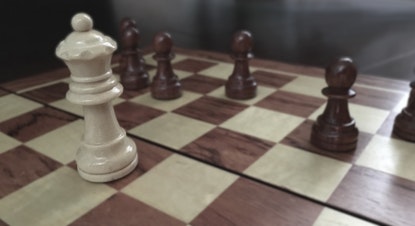
With over 15 government agencies now releasing procurement data to the Open Contracting Data Standard (OCDS), discussions at Open Contracting 2017 moved beyond how to publish to considering the quality of what was being released and how to maximize its use.
The OCDS schema provides a structure for what and how to publish data. Although this common structure makes it possible to compare datasets, the schema itself doesn’t say much about how complete or “good” those datasets should be.
There are now many, many thousands of contracting processes published in OCDS format, allowing us to start asking questions about what the data contain. But just using the OCDS format is not enough. Data that are sparse, of poor quality, or that does not meet user needs for monitoring and measurement are not going to help an end user achieve their objectives. In the worst case, there is a risk of ‘openwashing’ – data having all the form, but none of the function, of transparency.
In a recent blog post, Jiri Skuhrovec, Datlab team member and DIGIWHIST project collaborator, put this challenge starkly:
Data can be OCDS valid without containing any information relevant to the contract.
Jiri proposes an approach with two pass-fail tests in order to receive an «OCDS stamp,» with additional letter grades for meeting additional criteria. The general idea of assessing OCDS data quality is one that a number of people are exploring and is really important.
The OCDS documentation contains our own early pilot model for thinking about the information and content within OCDS data, with a set of ‘publication levels’ described as ‘Basic’, ‘Intermediate’ and ‘Advanced’. These were initially introduced to help provide a step-by-step approach to data publication, recognizing that providing all the fields users might want may take a few iterations. This fits with the idea of open contracting as a journey, not a destination. As the updated implementation pages on the open contracting website try to show, making open contracting part of “business as usual” of government rather than a “bolt-on” will involve a number of cycles of learning and adaptation.
With these ideas of basic, intermediate and advanced levels remaining in our standard documentation, we’ve seen demand from publishers to benchmark their data against these – and that has created challenges. To be frank, the assessments that we have carried out under this framework are not all achieving the results we desire. They may incentivize “transparency for transparency’s sake” and box ticking as publishers seek to provide fields to reach a level – without thinking about how those fields are useful, or whether the contents of those fields are meaningful. This risks undermining the use-oriented model we want to support. This point was well illustrated in our recent work on use cases (see below).
So, in our conversations with partners, we’ve started to explore ideas for how to restructure our tools, assessment methodologies, and other resources to consistently promote the progressive publication of high quality and usable data.
What might an updated open contracting approach to data quality look like?
We want to see OCDS as the foundation for quality data: to provide methods and tools layered on top of the OCDS schema that help publishers get there and help users negotiate for the data they need for changing business as usual.
One common methodology for assessing quality is through ranking. With a ranking, one can directly compare datasets either on a single score or in some cases, across a range of dimensions. This makes it possible to see which datasets already perform well against a set of common metrics, and which demonstrate the greatest opportunity for improvement against those same metrics. There is evidence that government rankings motivate behavior changes, as Christopher Wilson describes in a recent literature review. At the same time, as he points out, rankings can promote adverse behavior: «Comparative and numerical global assessments can incentivize box checking rather than actual norm adoption.» And most rankings measure against some externally defined metrics, shifting power to those who set the general metrics, and away from the grassroots data users. So, the pros and cons of ranking must be carefully weighed.
Another approach may be to provide a flexible framework, that offers quality feedback relative to the particular use-cases for a dataset. An important consideration for this kind of data quality tool is defining which elements of data are the most important for which end users and then identifying how to assess the quality of those data elements. Here, we see an interesting trade-off between quantity of fields and quality: when it comes to data quality, more data doesn’t always translate to better data. We explore this balance in «Using it, not losing it, over procurement data», the latest addition to our use case guidance series. This new guidance includes:
- a research report on what five open contracting champions consider to be priority data, information, stakeholder, and publication requirements;
- a use case to OCDS mapping that suggests almost 50 new indicators each related to specific use cases (which compliment the 120 red flag indicators we identified in our previous round of research); and
- a guidance document for reading the mapping and diving deeper into how data can support the achievement of targets.
Next up, we want to engage more deeply with the open contracting community to provide richer guidance on what we mean by “data quality” and how actors across sectors can find real value in open procurement data. We are already thinking through a new set of guides, tools, and other resources regarding data quality that will hold community engagement at its core. Through this investigation and development process, we aim to find automated, reusable ways to provide data publishers with feedback on data quality and to support and incentivize publishers to identify and close information gaps.
Developing a clear methodology to assess data quality, and crafting the tools to operationalize it, is going to be a challenge and one that we are going to need a lot of help with. If you’re taking the time to read this blog post, we want you, whatever your level of technical expertise, to be included in our work on data quality.
We would love your feedback and thoughts so that our development process can be as community-oriented as possible (please send ideas to kwikrent@open-contracting.org). In the coming months, we will also be preparing more formal opportunities for organizations to propose and build data quality tools to assist our community to continually assess the scope and quality of OCDS datasets.
We would love to hear your real-world examples of where data quality assessment, especially automated assessment, has been done well elsewhere. If we can learn from these assessment models and create even stronger tools and guides to support the community, we can help set a wider precedent for the challenge of opening up the data that really matter in formats that encourage use and reuse. We can model the behavior that citizens around the world deserve from their government.