Let’s get real about AI’s potential to end corruption in procurement
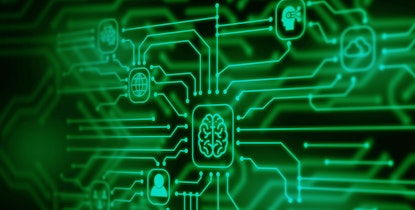
There is no question that preventing corruption is one of the primary goals of every public procurement system, as enshrined in the UN Convention Against Corruption. Traditional anti-corruption tools and efforts—including adequate legislative frameworks, oversight and enforcement bodies, and even the rising activism of civil society—increasingly work together to ensure probity in the spending of public funds. However, there are great difficulties in achieving anything close to ‘zero corruption’ in procurement despite such measures. The pandemic has brought a stinging (and expensive) reminder that procurement corruption is a significant risk everywhere, all the time, but especially in times of crisis.
The idea of using artificial intelligence (AI) to contribute to the anti-corruption effort in procurement is attractive. Enthusiasts go as far as arguing that digital technologies are changing the ‘corruption game’. The (slowly) growing availability of procurement data—especially as a result of the growth in adoption of the Open Contracting Data Standard (OCDS)—indeed brings with it the potential to deploy AI to combat corruption in procurement. AI could assist in automating anti-corruption screens based on set rules; in cross-checking data and interlink as of now disjointed databases of anti-corruption-relevant information; and in the longer term, training algorithms to generate corruption risk indicators on the basis of their own unsupervised learning of how procurement markets and environments operate. However, the possibility of developing an AI-based all-knowing anti-corruption ‘big brother’ capable of automatically and autonomously detecting and sanctioning corruption in public procurement simply remains science-fiction. More generally, the possibilities to harness AI functionality to fight corruption need to be assessed realistically and the costs and difficulties inherent to their development and implementation need to be recognized before policy makers jump on the bandwagon of pledging significant investments in this novel area of digital public governance.
In a recent paper, I argue that the expectations around the deployment of AI as an anti-corruption tool in procurement indeed need to be tamed. I offer four reasons for this:
- the deployment of AI for anti-corruption purposes is an information or data-processing based endeavour that can only rest on narrow and clearly defined tasks;
- there is still very limited procurement and other relevant anti-corruption digital (big) data and that, even if the implementation of current initiatives on open procurement data (such as OCDS) were maximized, they would still fall short of generating the necessary data architecture for comprehensive anti-corruption checks;
- ‘new’ data will be insufficient, but digitizing ‘old’ data is not a straightforward solution because, by the very nature of corruption (not always observable, and certainly not always detected and sanctioned), there are important questions looming over the quality of datasets; and
- there are significant legal constraints, as well as new governance risks inherent to using AI.
In the rest of this post, I provide a summary of the analysis behind these reasons (more details available in the paper), as well as the two conclusions I think are relevant to policy makers considering the deployment of AI-based anti-corruption solutions in a procurement context.
AI can only carry out narrow, information-based tasks
First, the functions that AI can carry out need to be well understood. Even if, under the right conditions, AI can be faster, more consistent and more accurate than human decision-makers at spotting instance of corruption, most narrow AI applications (e.g. robotic process automation of anti-corruption checks, or machine learning aimed at predicting corruption risk) cannot perform cognitive functions that humans would not also be able to carry out given sufficient time. From an anti-corruption perspective, AI can make positive contributions to the extent that it can: find or aggregate information that was previously inaccessible or costly to gather for human officials; cross-check information in ways that were not possible or were (too) time-consuming to carry out; (continuously, more quickly) run deviation checks against clearly established benchmarks (e.g. in the context of contract implementation, to ensure that the contracted outputs are delivered as originally established, to measure delays or raise flags around contractual modifications) or standards (e.g. legal or best practice standards) to identify anomalies or abnormalities in key (performance) indicators; or reliably classify specific procurement activities on the basis of their corruption risk. As such, AI can contribute incrementally to current anti-corruption efforts in procurement, but it cannot significantly alter (or substitute for) existing oversight and enforcement architectures. To put it simply, AI can deliver more screening for potential corruption and discharge human officials from that administrative burden so they can reorient their efforts to more value-added activities, but that screening cannot be based on rules or information that would not be available to a human anti-corruption official.
AI can only do so to the extent that quality data is available
Second, the extent to which AI can deliver such improvements is highly dependent on existing limitations in procurement data availability and quality. Given that the deployment of AI for anti-corruption purposes is an information or data-processing based endeavour, the existence or not of adequate sources of procurement data is the main constraint on its practical adoption. The crucial aspect is to ensure that relevant actors have access to the information they need, which raises the question whether current efforts—including the adoption of OCDS—would suffice. In my view, despite the increase in generation, recording and publication of procurement that comes with it, the current version of the general OCDS standard leaves out significant amounts of relevant anti-corruption information. This is particularly clear in comparing the general with the OCDS standard for Public-Private Partnerships (PPPs) currently being developed. This newer and more demanding standard would contain useful anti-corruption relevant information currently missing in the general standard, such as information on the qualitative selection of bidders and any exemptions granted from the standard requirements, decisions on conflicts of interest declared or uncovered during the tender, and decisions on any debarments (or exclusions) issued.
But even then, relying on the OCDS alone would be insufficient because it does not capture important information, such as the corporate linkages between economic operators, indirect control and conflicts of interest resulting from beneficial ownerships structures (although there is now a data standard for beneficial ownership information that can be linked to the OCDS), political connections (including political donations), revolving doors and other mechanisms of corruption (such as the use of common financial intermediaries or legal advisors) to name but a few. Perhaps one of the most promising anti-corruption uses of AI lies in the possibility of cross-checking procurement data with other sources of information. Which of course requires for those sources of information to also be available in an adequate format and curated in a way that ensures its quality and reliability. While some of those sources of information will also be held by the public sector and, as such, are susceptible of digitalization through open government initiatives beyond procurement, other sources may require public-private cooperation to generate adequate data exchanges, which can limit the extent to which a sufficient level of (digital) data availability can be reached so that anti-corruption AI solutions can be properly deployed.
‘New’ data will be insufficient, but digitizing ‘old’ data is not a straightforward solution
Third, there are also important issues on data quality and implicit biases in the data that need careful consideration. While some basic applications of AI (mainly, robotic process automation) can be deployed as soon as procurement databases start being available to automatically retrieve information from them, their added value will be limited to the extent that they can only rely on newly (automatically) generated information. Unless previous procurement (and other) records are digitized and made available for the purposes of data retrieval and cross-checks, the value of these implementations will only arise with a significant time lag (in other words, we will have to wait for the OCDS or otherwise automatically generated data to get sufficiently big).
However, the use of pre-existing information to accelerate the uptake (and value generation) of AI anti-corruption solutions creates some additional problems. One of the concerns can be the cost and the time required to create such databases. And, pragmatically, a decision could be made to only digitize samples of records or records for a limited period of time (e.g. the last three years). However, these decisions need to be carefully considered against the biases that they could generate in the data, as creating unbiased datasets based on sampling or short periods of time can be exceedingly complicated.
Another difficulty in creating unbiased datasets on which to train algorithms is the massive challenge of assembling a sufficient and high-quality database of corrupt and non-corrupt procurement exercises from which the algorithms can learn. The difficulties here stem not only from the long time it can take for (criminal) corruption cases to be legally final (which creates uncertainty in the data, at least for suspected corruption cases), but also from the fact that corruption is not detected in 100% of the instances and that it is very hard to assess its prevalence, which practically means that cases that could be considered ‘clean’ in a database may in reality represent undiscovered instances of corruption.
All of this means that past data needs to be reassessed very carefully and probably often discarded, even if only to avoid training algorithms that entrench screening approaches unable to detect sophisticated or difficult to observe corrupt practices.
There are significant legal constraints, as well as new governance risks inherent to using AI
Fourth, any discussion of the potential uses of AI for anti-corruption efforts must consider the legal framework on which it is to be deployed. From that perspective, the existence of legal constraints on administrative (and criminal) law decision-making need to be considered as a further layer of regulation conditioning the potential use of AI for anti-corruption efforts in public procurement (and more broadly).
Even if every jurisdiction has its regulatory specificities, common constraints will derive from: the right to good administration, which will imply both a duty to rely on accurate information and to assess it objectively as a prior step towards adopting a decision, and a duty to provide reasons in a way that is meaningful to the addressee of a given administrative decision; as well as the right of access to justice, which will generally imply the right to challenge a decision that negatively affects one’s individual rights.
These will be relevant in relation to all types of implementation of AI for anti-corruption purposes, but also increasingly relevant as the information or indicators generated by the AI inform corruption-related administrative and criminal law decision-making.
I argue that a human decision-maker will necessarily remain in the loop and this, rather than reducing good administration and due process concerns, both exacerbates them and creates new corruption risks.
Firstly, it will be necessary to develop additional layers of oversight to tackle the AI-human interaction and, in particular, to avoid over-reliance on AI recommendations through ‘dead-driver’ vigilance devices, to prevent the mindless rubber stamping of AI-generated proposed decisions, and to avoid undesirable feedback loops and perverse dynamics in the adjustment of the algorithms supporting human decision-making that could result from failing to correct faulty AI outputs.
Secondly, the fact that a human decision-maker needs to remain involved simply implies that such an individual will be exposed to corruption risk in a similar manner as they were before the deployment of the AI solution supporting their decision-making. Therefore, it will be necessary to ensure the continuation of the controls applicable to human decision-making in corruption-prone activities.
Implications for policy making
The analysis leads to two valuable implications for policy makers.
First, prioritizing improvements in procurement data capture, curation and interconnection is a necessary but insufficient step. The gains that can be derived from such efforts, while considerable, are insufficient to tackle less obvious forms of corruption.
Taking corruption in procurement seriously requires a broader and more comprehensive approach to the digitalization of other databases, both public and private. However, there can be significant constraints resulting from the ‘open access’ approach to current procurement data initiatives, such as OCDS.
I argue that increasing the availability of the relevant information to the adequate actors does not necessarily mean that all this information should be made public in real time, or in full. Policy makers may want to consider how developing more nuanced approaches to open data can contribute to further advances in the deployment of AI for anti-corruption purposes, at least in jurisdictions where resistance to digitalization and data interconnection or exchange stem from concerns about public overexposure. Tackling the issue in a more comprehensive manner may require recalibrating, for example, freedom of information rules concerning some data, as well as boosting in-house capabilities to develop anti-corruption tools in countries that may be over-reliant on civil society interventions.
The second implication of the previous analysis is that existing anti-corruption oversight and enforcement architectures need to be maintained or even expanded, and that there is a need to ensure that the training and upskilling required to make use of AI solutions does not come at the cost of core capabilities. Investment in anti-corruption AI cannot be seen as a substitute for traditional investment in these efforts because existing and foreseeable AI solutions can act as a complement, but not a substitute of current approaches to the prevention, detection, and sanction of corruption in public procurement. Moreover, the deployment of AI solutions for anti-corruption purposes in the procurement context (and more generally) creates its own governance and corruption risks, and these too require the development of additional layers of monitoring and oversight that will need resourcing.
It is possible that, in the long run, the deployment of AI solutions can contribute to a reduction of the overall level of investment and resource required by anti-corruption oversight and enforcement architectures, but this will only be the case to the extent that the pre-AI system is disproportionately investing time and effort in the specific information and data-processing activities that can be automated—compared to higher value-added investigative and decisional activities. However, even then, in the short to medium term, it seems likely that the development of AI-supported capabilities will require an expansion in the volume of resources dedicated to combating corruption in public procurement.
Prof Albert Sanchez-Graells is a Professor of Economic Law and Co-Director of the Centre for Global Law and Innovation, Law School, University of Bristol (UK).